iPhones on Wheels
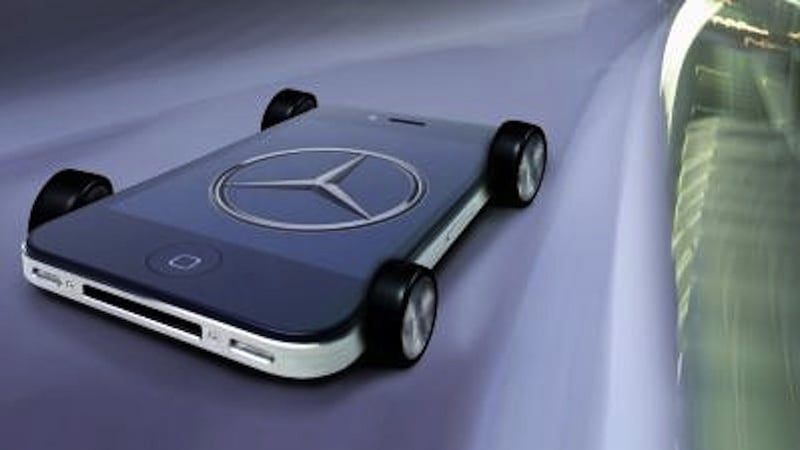
Modern road vehicles are full of computers, and getting fuller. In fact, the factors driving the digitisation of vehicle systems are familiar for many other technology developments, namely safety, efficiency and power. A brief look at the second century of automotive brakes shows the digitisation of braking systems has been with us for nearly 50 years, and the growth in importance of digital devices to a system as fundamental as braking should underline how deeply integrated software-related systems are now to mobility.
Indeed, the great silicon shortage of the 2020s has shown that there are very few corners of industry that are untouched by digitisation. Smartphone makers may be able to pay more to overcome such disruption, but Auto makers don’t have such generous margins to leverage, and therefore have to wait their turn. And while this effect has been felt in many corners, it is likely to cast a longer shadow on automotive production, highlighting just how important silicon has become to land transport.
So now that our cars are full of chips talking to each other, how much digital chatter is going on? Well, with the advent of external sensors in our vehicles, the answer is quite a lot. A vehicle with L2 ADAS may generate 25GB/h while a contemporary autonomous vehicle may generate between 1.4 and 19TB/h. It’s worth saying that these estimates vary widely, and there is no uniform comparison standard. But modern vehicles gather and process a lot of data, and OEMs are having to get into the data warehousing game.

Vehicle DoJos
This digital vehicular discourse is radically changing the nature of how vehicles are created, driven, used and existed with. At its recent AI Day public event, Tesla disclosed some of the latest approaches it is taking in its quest for self-driving. Robot butlers aside, the investments in machine learning, proprietary silicon design and data processing scale are jewels that an automotive company from even a decade ago would have struggled to justify, let alone push to the forefront of their crown.
While data as the new oil may not be the case in every automotive discipline, the definition of a driver is certainly a rich field. Beyond the on-board driver aids and mimics previously mentioned, insurance for drivers and their cars is being transformed by data mining. The provision of any insurance is, by definition, a probability problem, and no more so than for road use. The large lakes of data generated by our driving can be harnessed to create more intimate and detailed profiles of individual drivers, and better definition of the traffic conditions we navigate. How we use our cars in traffic directly relates to the energy stores we consume, and here AI is being applied to make more informed decisions on both the streets we take and the power deployed in moving through these streets.
Chassis and dynamic applications
So taking a closer look at how data is used in chassis systems, let us start with some of the more straight forward applications of data and location to improve driving performance. Among these is the simple idea of matching a ride height recommendation to a location for vehicles equipped with ride height adjustment, based on previous adjustments. A similar concept allows for predictive braking and energy optimisation based on known route topology and intersections.
Next is the idea of sharing pooled data (so-called swarm data). This can assist vehicles to better understand skid hazards on a route or location to improve stability performance. While swarm data is nothing new (think Yelp, TripAdvisor or even Waze), bringing in detail about individual lanes or hazards for more precise driving decisions is.
Focusing in on brakes, then, an area where significant data is generated during development is Brake NVH. Typically, during a brake system development, a manufacturer will run multiple pre-production vehicles over set routes, in an attempt to screen for brake noise and wear patterns over the typical disc or pad lifecycle. Traditionally this has meant NVH datalogging with manual snapshot triggering once the accessor hears an event. Modern tools can be pre-programmed to trigger if signals exceed dB thresholds, but the projects still rely on “a tuned ear” being available, and assessments being collated.

At EuroBrake 2021, two new approaches to leveraging data science to brakes NVH were presented. Firstly, Dr. Merten Stender from Hamburg University of Technology presented an approach to predicting brake squeal using deep learning. Dr. Stender’s team leveraged the large volume of available brake test data to detect and characterise vibrations in microphone measurements recorded during testing. Next, they created a data model of a brake system, a so-called digital twin, taking a sequence of loading conditions (e.g. pressure, deceleration, temperature, ambient conditions) as inputs and outputting a probability whether this braking scenario will exhibit a squeal noise. This model or twin was then tested with unseen data, and showed a high success rate in predicting brake squeal based on the unseen vibration data. The virtual twin is also capable to reliably predict other noises, such as wire brush and pad click. Readers will be interested to know that Dr. Stender’s recently founded a company, tensorDynamic, to exploit his AI concepts.

The second approach, presented by Fabio Squadrani of Applus Idiada, leveraged the unique database of NVH durability testing conducted by the experts of Idiada over many decades. Their novel approach combined the extensive collection of visual spectrographs from their NVH testing with image recognition tools to identify and categorise noise events based on the observed frequency, intensity and duration. This created a model which could then take unseen spectrographs, and identify the NVH issue present with a high degree of accuracy.

This solution is particularly appealing as it is built upon and integrated with Idiada’s current market-leading know-how in brake durability testing. As the model is trained and verified by the same experts that carry out this work across multiple customer vehicles in a wide variety of global locations, the solution offers rigour, robustness and flexibility. This AI approach will be integrated within the current Idiada service offer, and validated for a wider variety of use cases, as well as different high frequency noise types. Readers interested in learn more should contact the Idiada Brake Engineering team, and follow their presentations at the SAE Brake Colloquium.
Leveraging AI Allies
Both approaches show real potential to make significant strides towards virtual engineering in this area, compressing development cycles and freeing up development resources. Brake systems would be characterised by screening tests, but durability performance could be established via a digital twin, and sensitivities and countermeasures identified.
Dr. Stender also suggests that applied AI in this field would elevate data from its current short-lived role of assisting screening into a valuable ally in real-time noise suppression. By being able to successfully identify the brake conditions that trigger squeal, it’s possible to modulate pressure in the caliper, thereby preventing the squeal trigger. Furthermore, its possible to learn the individual habits of a user, and better tailor predictions and interventions based on this.
Hello? Is that the future calling?
And such approaches are not confined to brake noise. Applied AI techniques promise similar outcomes in areas such as emissions controls, predictive stability interventions, learning calibrations and personalised safety systems.
In many ways, todays chassis development techniques are reminiscent of the dumb phone era (all software finished the day the product ships, proven by exhaustive testing), whereas increasingly vehicle systems are embracing the smart phone paradigms of OTA updates, localisation, beta releases and user profiles. User expectations are changing, and the value propositions for OEMs are changing as well. It’s not enough to just ship a great product, the product needs to adapt to its users, often in ways that can’t be directly tested or quantified by the developers. OEMs may struggle to make the leap from their current cost and profit structure (cost intensive pre-production effort, single large profit per production unit) into the services model available to digital devices. However the significant costs of owning a running a vehicle mean there is significant value to be derived from harnessing vehicle data. Making smarter choices about energy flows, power demands, pre-emptive safety and security interventions and maintenance patterns means costs and risks are reduced. Harnessing dynamic vehicle data offers powerful toolsets to unlock these benefits.